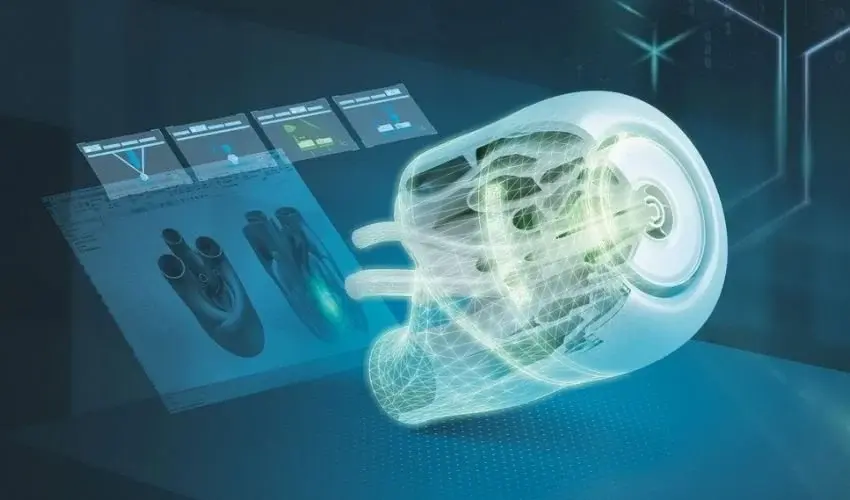
In additive manufacturing, artificial intelligence has become increasingly prevalent. Software developers and research institutions are actively harnessing machine learning's predictive capabilities in two main areas.
Process control is a critical aspect of industrial 3D printing. Despite significant technological advancements, challenges such as part defects, particularly porosities, remain prevalent. To address these issues, machine learning algorithms play a pivotal role. They are employed either for detecting defects or adjusting printing parameters, or sometimes both. This application aims to minimize print failures and optimize efficiency by conserving time and materials.
AI also plays a significant role in the development of innovative materials for 3D printing.
AI Role in 3D Generative Design Solutions
3D printing stands as a complex yet promising technology, poised for significant advancement with the integration of artificial intelligence (AI). One pivotal area where AI is making strides is generative design. This innovative approach harnesses machine learning algorithms to optimize 3D models effectively. Autodesk's Netfabb solutions exemplify this synergy, leveraging AI to generate diverse design solutions based on specific benchmarks provided by designers or engineers. These benchmarks can encompass various criteria such as product dimensions, weight, strength, style, materials, and cost.
Artificial Intelligence for Developing 3D Printing Materials
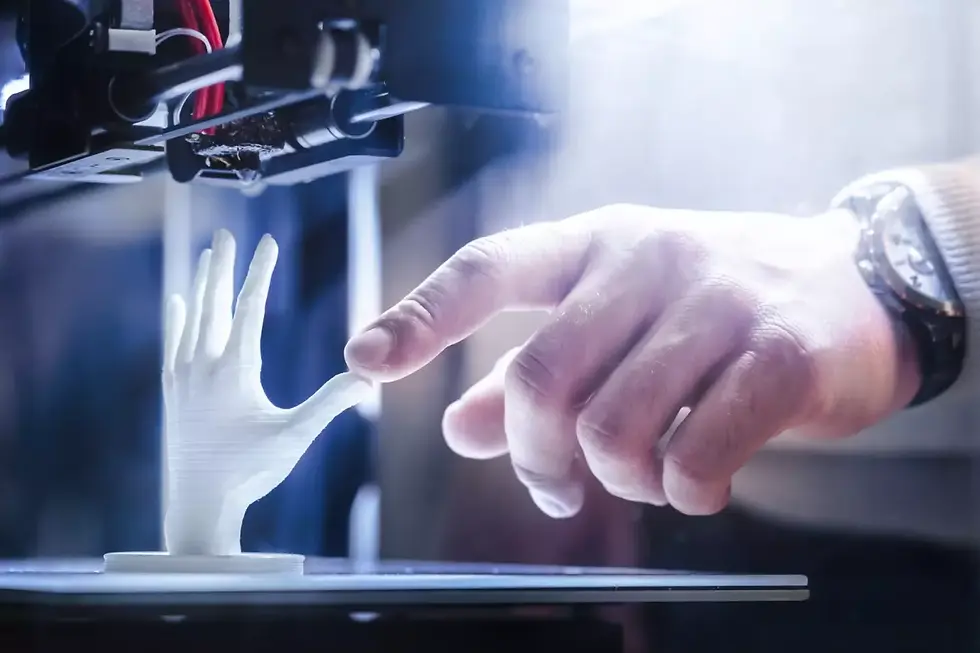
Formulating new materials is a complex endeavor, often involving extensive and costly testing to ensure optimal performance. Traditionally, this has meant relying on data crunching to correlate specific treatments or additives with desired material properties. However, the emergence of AI-driven solutions, such as Intellegens' Alchemite platform from the University of Cambridge, is revolutionizing this process.
Intellegens leverages machine learning to rapidly design materials tailored for 3D printing. For instance, they successfully developed a nickel-based alloy for Direct Laser Deposition by harnessing Alchemite's deep learning capabilities. This approach enabled them to extrapolate crucial alloy processability insights from a vast thermal resistance database with remarkable efficiency—only ten data points were needed. This showcases AI's potential to accelerate material innovation by pinpointing intricate property-to-property relationships swiftly.
Similarly, researchers at New York University's Tandon School of Engineering have utilized AI tools to reverse engineer complex 3D printed components made from materials like glass and carbon fiber. By applying AI algorithms to analyze CT scans of these parts, they could predict key properties such as structural strength and durability. This method allowed them to reconstruct components with an impressive accuracy of 0.33%, highlighting AI's role in enhancing manufacturing precision.
However, the integration of AI in material development and manufacturing is not without challenges. Concerns have arisen regarding intellectual property security, particularly with 3D printed composite parts. The ease with which AI algorithms can decode and replicate manufacturing processes underscores the importance of robust IP protection strategies in this evolving landscape.
Looking ahead, AI promises to further revolutionize material science, paving the way for advanced closed-loop control systems and innovative materials. The potential future integration of component-based search engines, capable of predicting ideal STL files for DIY projects, illustrates AI's expanding role in empowering both industrial and personal manufacturing endeavors.
In summary, while AI presents immense opportunities for accelerating material innovation and enhancing manufacturing efficiency, it also necessitates careful consideration of ethical and security implications. Embracing AI responsibly is crucial as we navigate towards a future where intelligent technologies shape the forefront of material science and production.
Comments